I. INTRODUCTION
Food security has always been recognized as a strategic imperative, ensuring the fulfillment of people’s rights to an adequate quantity, quality, safety, and nutrition to actualize a healthy, intelligent, active, and productive human resource. As the primary dietary staple food for the Asian population, rice has assumed a critical role in the country’s economy, transcending mere economic dimensions to encompass social and political implications. For disadvantaged communities in Asia, rice constitutes a significant portion of their expenditure. Due to the volatility of rice prices, less privileged communities are unable to manage their consumption level at higher costs (Dawe & Peter Timmer, 2012).
Indonesian agriculture has intermittently achieved self-sufficiency in rice production. According to the United Nations Food and Agriculture Organization, Indonesia attained an impressive 90 percent self-sufficiency ratio in rice production and demand from 2019 to 2021, reflecting the recommendable resiliency of its agri-food system. While nearly every region in Indonesia cultivates rice, not all regions yield surpluses due to insufficient production to meet local consumption needs. Despite the progress in achieving a robust rice supply, rice prices in Indonesia have exhibited inconsistency. The average price of medium-grade rice in Indonesia between 2018 and 2022 has surpassed the highest retail price set by the Indonesian government in 2017 (National Food Agency of Indonesia, 2023). The elevated cost and unpredictable fluctuations in rice prices pose a significant threat to people’s ability to access food,particularly for the substantial number of Indonesians who fall into the categories of poverty and near poverty.
Indonesia stands out as one of the Southeast Asian countries with the highest rice consumption rates. In 2021, it was estimated by the International Rice Research Institute that Indonesians continued to consume over 100 kg of rice per person annually. This amount is considerably higher than those of other ASEAN members, especially when compared to Malaysia and Thailand, which are just 99 kg and 81 kg, respectively (Patunru & Ilman, 2019). However, due to the high price of rice, most Indonesians, especially those from impoverished backgrounds, face significant challenges (McCulloch, 2008). As of September 2022, approximately 23.36 million people, equivalent to 9.57 percent of the Indonesian population, were identified as poor (BPS-Statistic Indonesia, 2023).
In terms of the overall national rice policy, the rice price policy assumes a crucial role. Understanding the dynamics of policy changes made by different administrations and their impact on the availability of this staple food represents a treasure trove of information in comprehending overall food policy and national food security. Ensuring stable and affordable food prices for consumers necessitates a focus on food pricing policy. However, the implementation of an effective food price strategy becomes increasingly challenging in the era of market globalization and limited government investment in development. Rice price stabilization in Indonesia has been entrusted to BULOG since the 1970s through the management of rice stock.1) Undoubtedly, this strategy underscores the necessity of a substantial government budget. Given the significant financial resources allocated to stabilize the supply and price of food, analyzing the factors and strategies of these measures in attaining desired goals becomes imperative.
II. LITERATURE REVIEW
The maintenance of domestic rice prices has emerged as a vital component of Asia’s developmental aspirations. Ensuring stability in staple food prices within economically disadvantaged nations serves as a safeguard against the perilous cycle of poverty forboth farmers and consumers. Moreover, it fosters an environment conducive to investment at the agricultural level, which, in turn, stimulates broader economic growth. By curbing the volatility in prices of other commodities and fostering social and political stability, this approach proves instrumental in encouraging investments throughout the economy. Given rice’s predominant role in the dietary patterns of the majority of Asians, coupled with the erratic fluctuations in the global rice market, all Asian nations have been compelled to fortify their domestic rice prices against international market fluctuations (Dawe & Peter Timmer, 2012).
The price instability of rice commodity causes a rapid shock response in the economy, namely changes in price that goes up and down which leads to inflation. Moreover, shifts in the economic sector are typically triggered by seasonal variations and natural calamities like floods, which can impede the distribution of goods when such disasters occur. Rice tends to be a risk during the rainy season due to many pests and fungus attacks, so yields are reduced and prices are rising. However, this does not make the demand for rice decrease because the demand will still exist to fulfill food needs (Aulia, 2022).
Indeed, the Asian nations that have achieved remarkable success in ensuring the nourishment and well-being of their populations have formulated their approaches around two key pillars within their domestic food systems, wherein they possess a certain level of policy authority: the rate of income expansion within the agricultural sector and the consistency of food prices (Dawe & Peter Timmer, 2012). The policy of price stabilization encompasses a wide range of instruments that incorporate measures such as storage facilities, subsidies, income supports, establishing minimum prices, and the utilization of rice distribution initiatives similar to Indonesia’s Rice for the Poor Program (Raskin), trade regulations such as tariffs, and quantity limitations have been put into practice. Among these diverse strategies, buffer stocks and trade policies have surfaced as the dominant approaches (Hoang & Meyers, 2015).
The holistic approach of implementing stock management through a public policy framework encompasses several interrelated components, including (1) the adoption of a floor price policy, (2) the implementation of a ceiling price policy, (3) the establishment of a domestic procurement policy, (4) the execution of a market operation policy, (5) the implementation of a government rice reserve policy, (6) the enactment of a routine distribution policy for revolving stock, and (7) the formulation of a stock disposal policy (Ruspayandi et al., 2022). Initially, the floor price policy was in place in Indonesia until 2002, at which time it was supplanted by a Government Purchase Price (GPP) policy. This shift in policy approach marks a distinct philosophical departure from the previous base price policy.The government’s purchase price policy assumes a more administrative role, serving as a guideline for BULOG in the procurement of grain or rice (Arifin, 2020).
Then, in 2017, the government established the highest retail price as part of the pricing policy for rice commodities. The program attempts to stabilize rice prices, minimize uncertainty for farmers, and ensure consumers have enough rice at a reasonable price (Isharyanto, 2018). The highest retail price is the price cap on whether the government needs to intervene in the market or not. When the price of rice surpasses a certain percentage of the highest retail price, it signals for the government to come down to the market to intervene through market operations.
Market operations are essentially policy instruments designed for controlling price increases. Market operations that increase the volume of rice on the market are projected to gradually lower rice prices. Initially, the amount of market operating rice was never intended, as market forces dictated. This is distinct from the current government’s market operation program “Supply Availability and Price Stabilization (SAPS),” which was launched in 2018 with a daily distribution aim. Is carried out without regard for market price changes, in contrast to prior market operations, which were carried out only if the price surpassed or was higher than the price that was set (Khudori, 2019).
In the countries that adopt such measures, the governments typically delegate exclusive authority to a State Trading Enterprise to monopolistically acquire rice or paddy for the purpose of establishing buffer stocks. This process commonly involves two distinct operations: domestic procurement and trade. Domestic procurement pertains to the seasonal purchase of rice or paddy by State-Owned Enterprises (SOEs) from farmers during the peak harvest period. Subsequently, the stocked rice is released during periods of low production. Moreover, the SOEs assess and regulate thevolume of imports and exports based on the availability of domestic supply and the relative relationship between global and domestic prices. Consequently, it results in the imposition of quantitative restrictions on net trade, rather than allowing it to fluctuate in response to world prices (Hoang & Meyers, 2015).
The underlying theoretical framework supporting the implementation of rice price stabilization policy in Indonesia draws upon a price equilibrium approach coupled with an intervention model incorporating floor price and ceiling price policies. The effectiveness of this policy approach in Indonesia has been substantiated through empirical evidence (Ruspayandi et al., 2022). However, Aryani (2021) highlights that despite the influence exerted by market operations and Rice Imports (RI) on rice prices, their efficacy in achieving price stability remains limited due to constraints related to inadequate time allocation and insufficient quantity in policy implementation.
III. MATERIALS AND RESEARCH METHODS
The research methodology employed in this study encompasses a double logarithmic regression model analysis, Autoregressive Integrated Moving Average (ARIMA) analysis, and descriptive statistical analysis to comprehensively investigate the factors influencing rice prices at the consumer level, and measure forecast Consumer Rice Price (CRP) accuracy. The study utilizes monthly time series data spanning a period of five years, from January 2018 to December 2022. It is important to note that the data considered in this research commences in 2018, assuming the implementation of the highest retail prices policy and the discontinuation of rice distribution through the Raskin or Rastra programs.2)
The selection of research variables is based on previous studies involving different time periods, varying circumstances, and recent policy developments. This approach is expected to provide a different perspective and more contextually relevant policy recommendations for the future, considering the diversity of potential impacts within an ever-evolving environment. The retention of variables from previous research is guided by empirical evidence demonstrating their relevance and sustainability within the context of government policies.
The previous study conducted by Aryani et al. (2017) analyzed the influence of three variables Rice HPP, Market Operation (MO), and Rice Import on CRP. Market operation is defined as a combination of Pure MO/ “Operasi Pasar Murni” (OPM) and Special MO / “Operasi Pasar Khusus” (OPK). The current research is conducted using a different condition and definition of market operation, as the OPM program was discontinued in 2017 and subsequently replaced with a new program called Food Supply Availability and Price Stabilization. Therefore, it is essential to evaluate the effectiveness of this new program, as the previous program was deemed less effective.3)
Consideringthat Indonesia’s policy to stabilize rice prices is carried out by managing stocks and regulating international trade policies, it becomes essential to introduce a fresh variable referred to as the “Central Government Rice Reserve (CGRR)” referred to as the government rice reserve part of the government policies for stabilizing supply and price of food. Detailed information on the research variables, including their definitions, sources, and notation, is provided in <Table 1>.
The double logarithmic model analysis is a robust statistical technique employed to examine the relationships between a dependent variable and multiple independent variables. In this study, regression analysis was utilized to assess the impact of the independent variables (X1, X2, X3, …, Xi) on the dependent variable (Y). Through this analytical approach, the direction and magnitude of influence exerted by the independent factors on the dependent variable were determined. The equation model encapsulating the identified variables can be expressed as follows (Ghozali, 2018). The general equation based on the variable identification:
Description:
lnCRP : The natural logarithmic of the Consumer Rice Price (Rp/kg)
α : Intercept
βi : Regression coefficient to (i) (i= 1, 2, 3, 4) that measures the elasticity of the relationship between a dependent variable and an independent variable. The percentage change in X (independent variable) and its impact on the percentage change in Y (dependent variable). A positive βcoefficient indicates a positive relationship between X and Y, while a negative β coefficient indicates a negative relationship.
lnGPP : The natural logarithmic of Government Purchase Price (Rp/kg)
lnMO : The natural logarithmic of Market Operations (kg/mon)
lnCGRR : The natural logarithmic of Central Government Rice Reserve (kg/mon)
lnRI : The natural logarithmic of Rice Import (kg/mon)
e : Standard error.
This research presents a comprehensive analysis of double logarithmic regression, facilitated by advanced technology such as IBM SPSS 27.0 software (Chicago, IL, USA). The study encompasses various classical assumption tests, including the Normality Test, Autocorrelation Test, Multicollinearity Test, and Homoscedasticity Test.
The evaluation of the estimation model incorporates essential statistical tests such as the F-test and t-test to determine the influence of independent variables (X) on the dependent variable (Y). Furthermore, the examination of individual independent variables’ effects on the dependent variable is conducted through the t-test, while the combined impact of multiple independent variables is assessed using the F-test. To evaluate the model’s overall quality, the coefficient of determination (R2 test) was employed alongside the F-test and t-test, providing crucial insights for hypothesis testing and model assessment.
ARIMA is indeed one of the most popular models in time series forecasting. This model consists of three fundamental components: AR (AutoRegressive), I (Integrated), and MA (Moving Average). By integrating autoregressive, differencing (integration), and MA components, ARIMA enables researchers to identify patterns, trends, and fluctuations within data and make forecasts for future values.
Model ARIMA consists of three processes: autoregressive, integrated, and MA, denoted as ARIMA (p, d, q). The order ‘p’ represents the autoregressive process in the model, ‘d’ indicates the integrated process that must be applied to the data first, and ‘q’ represents the MA process. If ‘d’ is 0 and ‘q’ is 0, the model is denoted as AR (p) for autoregressive-only. Similarly, if ‘d’ is 0 and ‘p’ is 0, the model is denoted as MA (q) for MA only. When all three processes are present in the model, it is called an ARIMA,denoted as ARIMA (p, d, q). An ARIMA model can be an AR (p) model or an MA (q) model or a combination of that is ARMA (p, q).
The AR model (p) is presented in the following equation (1):
The MA model (q) is presented in the following equation (2):
The ARMA model (p, q) is presented in the following equation (3):
The determination of the best-fitting model in this research relies on the Mean Absolute Percentage Error (MAPE) parameter. Error, or residuals, represent the difference between actual observed values and their corresponding predicted values. The MAPE is determined by calculating the absolute error for each time period, dividing it by the actual observed value for that time period, and then taking the average of these absolute percentage errors. The resulting figure is then multiplied by 100 to express it as a percentage. This method is particularly useful when the magnitude of the predictor variables plays a crucial role in evaluating the accuracy of predictions. The formula for MAPE is as follows:
Description:
n : number of time series data points
Yt : actual value at period t.
Y′t : forecast value for period t.
Analysis of the model using the MAPE method can be evaluated within the MAPE value range as <Table 2>.
MAPE (%) | Interpretation |
---|---|
<10 | Highly accurate forecasting |
10–20 | Good forecasting |
20–50 | Reasonable forecasting |
>50 | Inaccurate forecasting |
Source: Lewis (1982).
This research is dedicated to the policy measures employed in Indonesia to maintain stability in the prices of rice, specifically focusing on key instruments such as the GPP, MO, CGRR, and Rice Imports. These measures are essential in ensuring stability in rice prices at the consumer level. However, these policies face diverse challenges, some of which are beyond the government’s control. By implementing appropriate and effective rice price stabilization policies, the desired goal of consumer price stability can be achieved. Furthermore, these policies have far-reaching impacts, including inflation suppression, maintaining stability in prices for both producers and consumers, managing government food reserve stocks, and providing consumers with access to high-quality rice at reasonable prices.
Hypotheses serve as temporary proposed solutions or assumptions for research issues that require empirical verification (Sugiyono, 2013). In this study, the hypotheses are formulated based on existing theoretical foundations and previous research, addressing the following aspects:
-
The GPP has a negative effect on CRP.
-
MO have a negative effect on CRP.
-
CGRR has a negative effect on CRP.
-
Rice imports have a negative effect on CRP.
This study presents a rigorous and formal analysis, grounded in established methodologies, to explore the hypothesized relationships and determine the factors and strategies of rice price stabilization policies, and the importance of CGRR. This study contributes to a deeper understanding of the complex dynamics associated with rice price stabilization policies and their implications for consumer price stability. The findings offer valuable insights to policymakers and stakeholders, guiding them in the implementation of effective policies and enhancing overall food security in Indonesia.
IV. RESULT AND DISCUSSION
The analysis of the Indonesian government’s strategies implemented by BULOG to stabilize rice prices at the consumer level from 2018 to 2022 has been conducted using double logarithmic regression. The double logarithmic regression model allows us to examine the relationship between the independent variables and the dependent variable, where changes in percentage in the independent variables lead to corresponding changes in percentage in the dependent variable.
To assess the accuracy of the sample regression function in estimating the actual value, the goodness of fit measures is employed. These measures include the coefficient of determination, the F-statistic, and the t-statistic. The results of the double logarithmic regression analysis using the SPSS 27.0 program are presented in <Table 3>, which showcases the values of the coefficient of determination, the F-statistic, and the t-statistic, providing statistical insights into the magnitude and significance of the relationships observed.
Based on the analysis results using the double logarithmic regression method, the estimated values for αβ1, β2, β3, and β4 can be formulated into the regression equation (4) as follows:
The analysis conducted in this study focuses on the relationship between the dependent variable, CRP, and several independent variables, namely GPP, market operations, CGRR, and RI. As presented in <Table 3>, the R-value is 0.811, suggesting a strong positive linear relationship between the variables in the regression model. The Adjusted R Square value is 0.632, indicating that 63.2 percent of changes in the CRP can be explained by these variables. The remaining 36.8 percent are influenced by other variables that are not included in this study. These findings suggest that the government’s implementation of a rice price stabilization policy through BULOG has contributed to regulating fluctuations in CRP by 63.2 percent.
The results of the F-test, shown in <Table 3> as an output of the analysis of variance (ANOVA), reveal that an F-value of 26.385 is higher than an F-table of 2.733. Additionally, the probability value of 0.000 is lower than the significance level of 0.050. These results indicate that the independent variables (GPP, market operations, CGRR, and RI) collectively and significantly influence the dependent variable (CRP) at a 95% confidence level. To assess the individual effects of the independent variables, the t-test was employed, and the statistical results are displayed in <Table 3>.
The t-value for GPP is –5.235, which is greater than the t-table value of 0.672, with a p-value of 0.000, which is smaller than the significance level of 0.05. Therefore, the null hypothesis (H0) is rejected, and the alternative hypothesis (Ha) is accepted. This means that at a 95% confidence level, GPP has a significant effect on CRP. The coefficient for GPP is –0.13, indicating that a one percent increase in GPP will lead to a decrease in CRP by 0.13 percent. This finding highlights the effectiveness of GPP as a tool for price stabilization and affordability for consumers. When the government increases the purchase price it offers to rice producers, it acts as an incentive for increased production, helping to ensure a stable supply of rice in the market. As a result, this proactive approach can lead to lower CRP, benefiting consumers and contributing to food security in Indonesia. In the context of rice price stabilization policies, GPP emerges as a key policy lever for the government to manage and regulate CRP effectively.
The t-value for MO is –5.830, which is greater than the t-table value of 0.672, with a p-value of 0.000, which is smaller than the significance level of 0.05. Therefore, the null hypothesis (H0) is rejected, and the alternative hypothesis (Ha) is accepted. This means that at a 95% confidence level, MO has a significant effect on CRP. The coefficient for MO is 0.01, indicating that a one percent increase in MO will lead to an increase in CRP by 0.01 percent. This result may appear counterintuitive, as market operations are typically employed to stabilize prices rather than increase them. The unexpected direction of influence suggests that the effectiveness of MO in controlling CRP may be subject to further examination. While MO is a recognized tool for price stabilization, the findings imply that a closer evaluation of its implementation and policies is necessary to align it with the goal of reducing or maintaining CRP.
The scheme of market operations serves as a government tool to create a balance among the interests of producers, consumers, and overall economic stability. The objective of these market operations is to influence the supply and demand in the market, ensuring that the price of rice commodities can be controlled or maintained at a level considered reasonable or stable. Market operations are executed by closely monitoring the developments in rice prices in comparison to the prices predetermined by the government as the highest retail price.
The t-value for CGRR is greater than the t-table value of 0.672, with a p-value of 0.000, which is smaller than the significance level of 0.05. Therefore, the null hypothesis (H0) is rejected, and the alternative hypothesis (Ha) is accepted. This means that at a 95% confidence level, CGRR has a significant effect on CRP. The coefficient for CGRR is –0.02, indicating that a one percent increase in CGRR will lead to a decrease in CRP by 0.02 percent. This implies that an increase in the CGRR serves as an effective policy tool in stabilizing and reducing CRP. In practical terms, when CGRR is bolstered, it can be used to release rice into the market, thereby mitigating any price surges and ensuring a stable supply of affordable rice to consumers.
The t-value for RI is –2.804, which is greater than the t-table value of 0.672, with a p-value of 0.000, which is smaller than the significance level of 0.05. Therefore, the null hypothesis (H0) is rejected, and the alternative hypothesis (Ha) is accepted. This means that at a 95% confidence level, RI has a significant effect on CRP. The coefficient for RI is –0.0004, indicating that a one percent increase in RI will lead to a decrease in CRP by 0.0004 percent.
This finding suggests that RI can be a valuable strategy for managing and stabilizing rice prices. When the government permits or facilitates RI during periods of domestic scarcity or rising prices, it can contribute to averting price spikes and ensuring an adequate supplyof rice for consumers. In the context of price stabilization policies, RI emerge as a key variable that authorities can employ to address fluctuations in CRP effectively.
CRPwere forecasted utilizing data obtained from the National Food Agency of Indonesia spanning from January 2018 to December 2022. Using this dataset, researchers generated predictions for CRP for the period from January 2023 to December 2023. The methodology employed for model determination and price forecasting relied upon the time series approach known as the Box-Jenkins method or ARIMA, implemented with the assistance of IBM SPSS 27. The ARIMA model is employed for the analysis of time series data exhibiting short-term trends, with the objective of generating future predictions. The ARIMA model will produce forecasts for CRPbased on the best-fitting model with the smallest MAPE values.
<Table 4> and <Table 5> present the results of the parameter values obtained from each ARIMA model estimation for CRP data. The determination of the best-performing model is based on the highest R-squared value and the lowest values for Root Mean Square Error (RMSE), Mean Absolute Error (MAE), Bayesian Information Criterion (BIC), and MAPE. According to the criteria outlined in <Table 5>, the ARIMA (1,0,2) model exhibits the highest R-squared value and the lowest RMSE, MAE, and MAPE among the five ARIMA models considered. Hence, it can be concluded that the best-performing model to be employed is the ARIMA (1,0,2) model.
The purpose of significance testing is to determine whether the parameters obtained from the ARIMA model are statistically significant. A model is considered significant if the significance level (p-value) of each parameter is less than alpha (α), with a value of α equal to 0.05. The model is also deemed significant if the significance level (p-value) indicates the presence of white noise in the residuals, which means that the hypothesis is satisfied. White noise in residuals occurs when the significance level (p-value) in the Ljung-Box test is greater than alpha (α), with the value of α equal to 0.05.
Based on <Table 4>, for the ARIMA (1,0,2) model, the significance level (p-value) of each parameter is less than α (0.05), and the significance level (p-value) in the Ljung- Box test is greater than α(0.05). This indicates that the residuals of the ARIMA (1,0,2) model exhibit white noise and are well-distributed. The ARIMA (1,0,2) model has satisfied the significance testing hypothesis and has passed diagnostic checks. Therefore, the ARIMA (1,0,2) model is considered appropriate for making forecasts.
Following the testing steps conducted, only one ARIMA model is considered as the best model for forecasting CRP, which is the ARIMA (1,0,2) model. The ARIMA (1,0,2) model underwent an accuracy assessment using the MAPE. The MAPE value is used to determine the accuracy of the forecast on the test data. <Table 5> presents the MAPE value for the ARIMA (1,0,2) model, which is 0.41%. This indicates that a forecast with a MAPE value of less than 10% is considered highly accurate forecasting, less than 20% is considered good forecasting, between 20%-50% is considered reasonably accurate forecasting, and a MAPE value exceeding 50% is considered inaccurate.
The Upper Control Limit (UCL) and Lower Control Limit (LCL) are boundary values utilized in ARIMA forecasting analysis to monitor forecast accuracy. UCL represents the upper limit indicating the highest level at which forecast results are deemed acceptable, while LCL represents the lower limit indicating the lowest level at which forecast results are considered acceptable. When forecast results fall within the range between UCL and LCL, it signifies that the forecast is within statistically acceptable bounds. However, if forecast results exceed the UCL, it may indicate over-forecasting or excessively high forecasts, whereas exceeding the LCL may suggest under-forecasting or forecasts that are too low. Therefore, UCL and LCL are used to monitor and identify inaccuracies in forecasting, aiding in taking necessary corrective actions to enhance forecast accuracy (<Table 6>).
<Figure 1> and <Figure 2> provide a comprehensive overview of actual CRP, predictions made by a regression model, and forecasts generated by an ARIMA model from 2018 to 2023. Over the period of 2018 to 2023, rice prices experienced significant fluctuations, as evidenced by the actual rice price chart. In early 2018, rice prices were at a certain level, and for the first few years, they continued to rise. However, around mid-2022, there was a significant price spike, followed by a sharp increase in mid-2023.
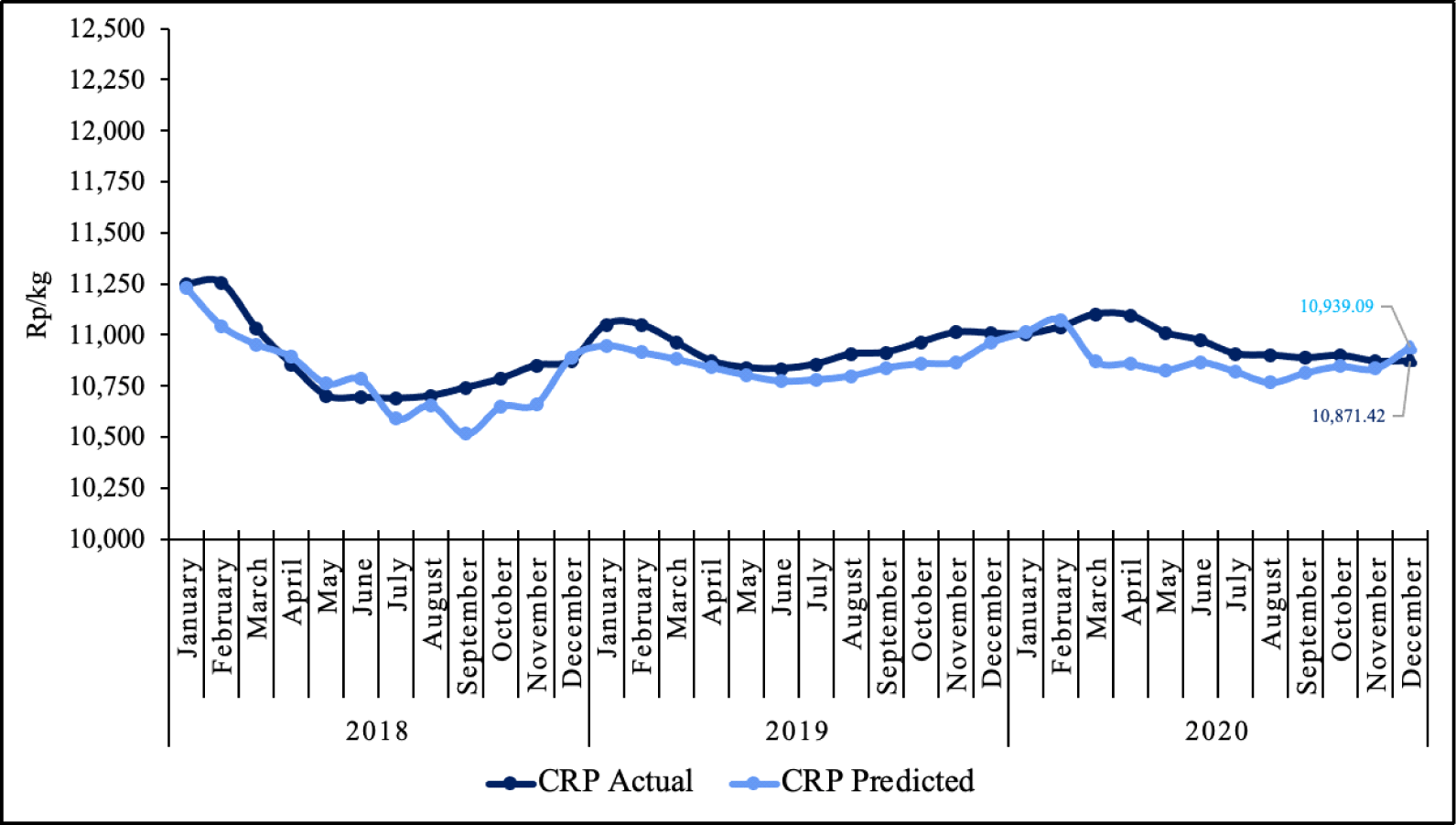
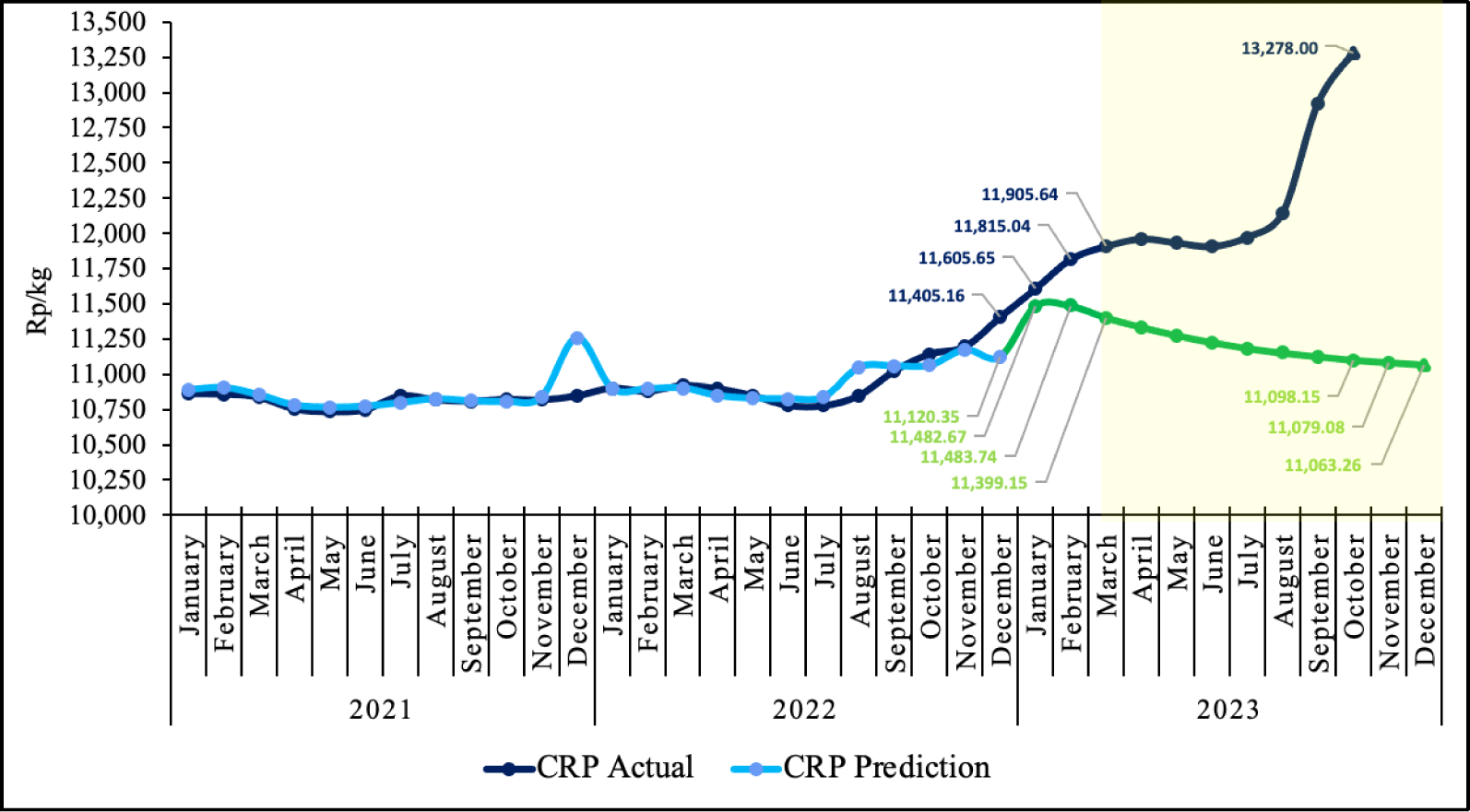
To gain a deeper understanding of these rice price trends, we applied double- logarithmic regression analysis. The results of the regression analysis indicate that rice prices are closely linked to various factors and government interventions in terms of rice price stabilization. Price predictions based on our regression model show a stable trend, with anexpectation of moderate price increases during the years 2018 to 2022. As for 2023, we conducted a forecast using an ARIMA model. The ARIMA forecast results suggest that rice prices are expected to remain within a relatively stable range, with limited fluctuations throughout the year.
By using both of these analytical methods, we aim to provide a more holistic understanding of rice price movements and offer a deeper insight into what may occur in the coming year. Reflecting on the past, present, and future conditions will undoubtedly be a consideration in determining the appropriate steps or strategies in price stabilization policies. This may involve maintaining existing government efforts such as the GPP policy, MO, Government Rice Reserves, and Rice Imports, or adopting new strategies that are of concern to the government.
Examining the three comparative graphs in <Figure 2>, the 2023 forecast graph appears to be in stark contrast to the actual data for 2023. This disparity is due to the ARIMA model’s forecast, which solely considers or utilizes time series data for rice prices without considering other policy factors implemented by the government. The difference between the forecasted rice prices and actual prices in 2023 can be explained by changes initiated in mid-March 2023. As per the graph in the yellow- shaded area, the Indonesian government made significant adjustments to the GPP and Highest Retail Price.
The GPP, previously set at Rp8,300 per kg in Bulog’s warehouses, was revised to Rp9,950 per kg, and the Highest Retail Price, for the medium-grade rice widely consumed by the Indonesian population and the focus of this study, increased from Rp9,450 per kg to Rp10,900 per kg. These changes undoubtedly had an impact on the evolving market prices. As seen in the actual rice prices in 2023, there was a continuous and drastic spike. The ARIMA model’s forecasting results for the short two-month period exhibited the same pattern as the actual data for 2023, with consecutive increases. From this, it can be concluded that the ARIMA model produced can accurately predict prices in the short term, with the caveat that periodic updates are necessary to account for changes or responses to government policies.4)
The effective implementation of the GPPpolicy starting from March 2023, followed by a significant and rather drastic change in prices, reflects the success of this policy in stabilizing prices. Price stability is often defined as prices that are reasonable and fair in comparison to excessively low prices. The adjustment of the GPPpolicy in tandem with modifications to the highest retail price policy for rice will induce changes and shifts in the stability of rice prices at a particular juncture. This scenario underscores the dynamic nature of price stabilization efforts and highlights the government’s responsiveness to evolving market conditions, thus contributing to ensuring that rice prices remain both reasonable and stable.
V. CONCLUSION AND POLICY IMPLICATIONS
In light of the results from the double logarithmic regression analysis concerning government interventions aimed at stabilizing rice prices in Indonesia, it was observed that all of the following factors exerted significant influence. The GPP and CGRR emerged as the most effective instruments, while the RI instrument had a relatively minor impact, and the market operations instrument was statistically less effective, requiring further evaluation.
Based on the MAPE criteria, the ARIMA (1,0,2) model has been identified as the best model for forecasting CRP in the future. The ARIMA forecasting model, when integrated with policy analysis, offers a holistic understanding of rice price dynamics, guiding policymakers in shaping effective price stabilization strategies to respond to evolving market conditions. The ARIMA model’s 2023 forecast, when compared to actual data, underscored its capability to predict short-term price trends, particularly in response to government policy adjustments.
The combination of double logarithmic regression analysis, considering various factors influencing rice prices, along with the ARIMAmodel used for forecasting future rice prices based on historical data, represents an effective approach for depicting therice price conditions, evaluating the effectiveness of government interventions in mitigating rice price fluctuations, and outlining the strategies necessary to maintain rice price stability in the future.
To enhance the effectiveness of price stabilization, the government should consider improving the precision of market operations programs, focusing on aspects such as quantity, quality, and timeliness. Recognizing the difficulties faced by economically disadvantaged communities in managing their consumption at higher rice prices and acknowledging the limited impact of market operations programs, we recommend that the government extends direct support to the underprivileged population through food assistance programs, with a specific emphasis on rice distribution.
The forecasting of rice prices is a crucial instrument for the government to anticipate rice price conditions in the coming years. The ARIMA model serves as an effective short-term forecasting tool. Therefore, periodic forecasting is necessary to ensure precision in policymaking and stabilization efforts. Considering the effectiveness of government strategies, such as GPP, CGRR, and RI in stabilizing prices, it is imperative to maintain and further develop these policies in accordance with Indonesia’s rice market conditions.